Tatyana Sharpee, Ph.D.
Professor at the Salk Institute for Biological Studies
Computational Neurobiology Laboratory
Integrative Biology Laboratory
Edwin K. Hunter Chair
Seminar Information
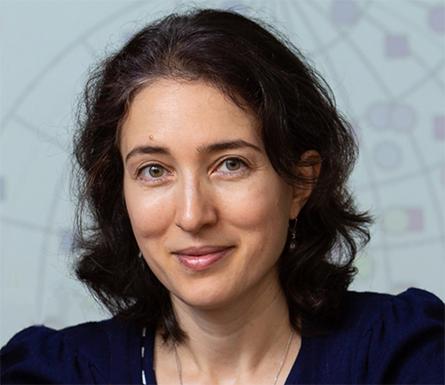
Across different scales of biological organization, biological networks often exhibit hierarchical tree-like organization. For networks with such structure, hyperbolic geometry provides a natural metric because of its exponentially expanding resolution. I will describe how the use of hyperbolic geometry can be helpful for visualizing and analyzing information acquisition and learning process from across biology, from viruses, to plants and animals, including the brain. We find that local noise causes data to exhibit Euclidean geometry on small scales, but that at broader scales hyperbolic geometry becomes visible and pronounced. The hyperbolic maps are typically larger for datasets of more diverse and differentiated cells, e.g. with a range of ages. We find that adding a constraint on large distances according to hyperbolic geometry improves the performance of t-SNE algorithm to a large degree causing it to outperform other leading methods, such as UMAP and standard t-SNE. For neural responses, I will describe data showing that neural responses in the hippocampus have a low-dimensional hyperbolic geometry and that their hyperbolic size is optimized for the number of available neurons. It was also possible to analyze how neural representations change with experience. In particular, neural representations continued to be described by a low-dimensional hyperbolic geometry but the radius increased logarithmically with time. This time dependence matches the maximal rate of information acquisition by a maximum entropy discrete Poisson process, further implying that neural representations continue to perform optimally as they change with experience.
Tatyana Sharpee received her PhD in condensed matter physics from Michigan State University studying under the supervision of Mark Dykman. After her PhD, she started to work in computational neuroscience at UCSF where she developed statistical methods for analyzing neural responses to natural stimuli, which exhibit strong correlations and non-Gaussian effects. These methods made it possible to reveal new adaptation processes in the brain by comparing neural responses to white noise and natural stimuli. Her independent research program has started at the Salk Institute for Biological Studies where she is currently a Professor in the Computational Neurobiology Laboratory. Her group analyzes principles of information transmission in the brain and within cells. Dr. Sharpee is a fellow of the American Physical Society.